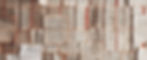
The volume and variety of digital content in organizations have grown many folds in recent years. Data exists in various formats – structured and unstructured, comes from multiple sources, and needs to be disseminated to a variety of channels. Managing digital content has become a laborious and challenging undertaking on many fronts. The process of sourcing, collating, organizing, classifying, enriching, normalizing, and distributing the data is often manual and gets increasingly complicated. Many content management platforms that help manage and maintain content are available in the market, but these platforms still require manual data entry and management and provide little automation. So the process continues to be laborious and time-consuming and prone to human errors.
The following sections of this article provide a high-level view of the capabilities and use cases of Machine Learning (ML) for content management. We discuss how Artificial Intelligence (AI) and Machine Learning (ML) can automate many steps of the content life cycle and help deliver better Data Products for customers. In subsequent posts, we will dive deeper into these use cases and provide guidelines for content processes in specific domains like eCommerce, Marketing, and Customer service.
Content Classification: The primary objective of content classification is to assign the correct hierarchy or class that the content belongs to. For example, Identifying the correct category of a product data taxonomy of a product, classifying a business policy document needs in the right security/access category, classifying social media posts by their aspects. Classification is essential for data organization for various reasons. From an operational perspective, it ensures the proper onboarding or handling procedures. From a customer’s perspective, it helps with surfacing the right content at the right time. From an information security perspective, it ensures appropriate controls are applied. This process usually involves using rule-based systems to classify content. The process is error-prone content and required regular rules maintenance. Machine learning models can help automate content classification and provide significant performance advantages over rule-based systems. Based on the historical data, these models can classify new content with minimal human intervention, significantly reduce classification errors and eliminate the rule-based systems.
Content Deduplication - Duplicate and conflicting content is a common problem businesses face as more and more data is ingested into systems. Companies often find themselves struggling with redundant, duplicate, conflicting, and obsolete information. Duplicate data can create many issues. For example, for an online retailer, inconsistent product information can result in pricing discrepancies or content that can confuse users. Inconsistent HR policy documents can confuse employees. AI can help manage content integrity and eliminate duplicate and conflicting information. Machine learning models for structured and unstructured data can be utilized to automate the data clean up and deduplication and normalization process.
Content Enrichment: The goal of content enrichment is to add or enhance the content for its consumers. The process involves enhancing descriptions, adding feature descriptions, or adding additional content or tags to improve the quality and discoverability of the content. For example, the distributors and retailers often get standard descriptions and attribute values for products from the manufacturers. To improve the differentiation, discoverability, and searchability of the products at their website or to get a higher-ranked result on web searches, the retailers enhance the descriptions of the product with their content and attribute additions. Content enrichment activities include:
Content Transformation - Transform the text to suit different target audiences or target specific user groups.
Content Tagging - Add tags to the content that help with the search, relevance, and affinity analysis.
Content generation - Generate new or alternate descriptions based on the current text.
Content Translation - Translate the content and its attributes from one language to another.
Several NLP-based AI models can help automate these activities and significantly reduce manual efforts.
We touched on the three common areas where AI can help organizations automate and streamline their content management process and improve efficiencies and data accuracy. In our subsequent articles, we will dig deeper into each of these areas to discuss the current AI methods and solutions and how to integrate them into your business processes and systems.